CTSA Program Support Enables Development of Life-Saving Blood Loss Monitor
December 6, 2019
A person can have dangerous internal bleeding but still appear to be healthy and stable, because the body compensates for blood loss by constricting blood vessels, taking bigger breaths, and making other changes to keep blood and oxygen flowing to the brain, heart, and other internal organs. These responses keep vital signs, such as blood pressure, from changing until a significant amount of blood has been lost. Because vital signs remain relatively stable despite significant blood loss, it often is difficult for medics and hospital staff to detect and appropriately treat internal bleeding before a patient goes into life-threatening shock.
As a trauma surgeon at the University of Colorado (CU) Anschutz Medical Campus and Children’s Hospital Colorado in Aurora, Steven Moulton, M.D., knew firsthand what this delay could mean for his patients. With support from the NCATS Clinical and Translational Science Awards (CTSA) Program hub at CU Anschutz, Moulton worked with researchers in the CU Department of Computer Science to find an innovative way to detect when someone is losing blood and how close they are to collapsing. The resulting device, called the CipherOx CRI M1, received approval from the U.S. Food and Drug Administration (FDA) in July 2018 and is now on the market.
"No one had been able to predict how close a patient was to collapsing," Moulton said. "So when we tell people we have a noninvasive method to trend acute blood loss—heartbeat to heartbeat—from normal to unstable collapse, they inevitably say, ‘You can’t do that, there is no such thing!’"
Uncovering Hidden Knowledge in the Data
In 2007, Moulton thought there must be information in patients’ vital signs that could provide an earlier warning signal of blood loss, but he needed a way to find that information and interpret it. He turned to two computer scientists at the University of Colorado and the Colorado Clinical & Translational Sciences Institute—Jane Mulligan, Ph.D., and Greg Grudic, Ph.D.—who were applying machine-learning techniques to enable robots to self-learn and navigate in unstructured outdoor environments. Moulton thought the same approach could be used to navigate the "unstructured data" from vital signs.
"At the time, people were working on this type of technology for autonomous vehicles but weren’t thinking about leveraging it to analyze vital sign waveform data in medicine," Moulton said. "But there are a lot of similarities between looking at raw visual data from a robot’s sensors and looking at noisy waveforms from vital sign sensors."
The team decided to use an unsupervised machine-learning approach in which a computer tried to group the data based on patterns without knowing anything about the data, such as which patients were losing blood and when they became unstable. This unbiased method could identify patterns from large amounts of clinical data that would be impossible for a human to see.
But first, the team needed data. Moulton contacted Victor Convertino, Ph.D., at the U.S. Army Institute of Surgical Research (USAISR). Convertino had brought a lower-body negative pressure (LBNP) chamber from NASA to the USAISR to mimic bleeding. Healthy volunteers lie with their lower body inside the chamber, and a vacuum pulls blood away from their upper body into their pelvis and lower extremities, thus starving the heart of blood flow and mimicking acute blood loss. Meanwhile, a sensor on the volunteer’s fingertip monitors blood oxygen levels and collects pulsatile photoplethysmography waveform data. Over time, the team applied their machine-learning approach to pulsatile waveform data collected from more than 200 volunteer LBNP subjects, which resulted in more than 2 million waveforms for analysis. This training data enabled a powerful set of feature-extraction and machine-learning algorithms to identify and learn how a select group of pulsatile waveform features predictably change from completely normal to collapsed (systolic blood pressure < 80 mmHg).
The result was a mathematical model of how much someone is bleeding and how close they are to collapsing. The model evaluates the shape of each waveform of blood at a fingertip and knows how that waveform changes when the person is bleeding. The output is a new kind of vital sign that indicates how close someone is to collapsing, which the team has termed the compensatory reserve index (CRI).
Validating a Pioneering Approach
Moulton and the team were excited, but a lot more work lay ahead before this technology could reach patients. Moulton received a CTSA Program pilot award in 2009—his first funding for the project—to develop the CRI algorithm and later port it to a small, battery-powered device that could be deployed on the battlefield.
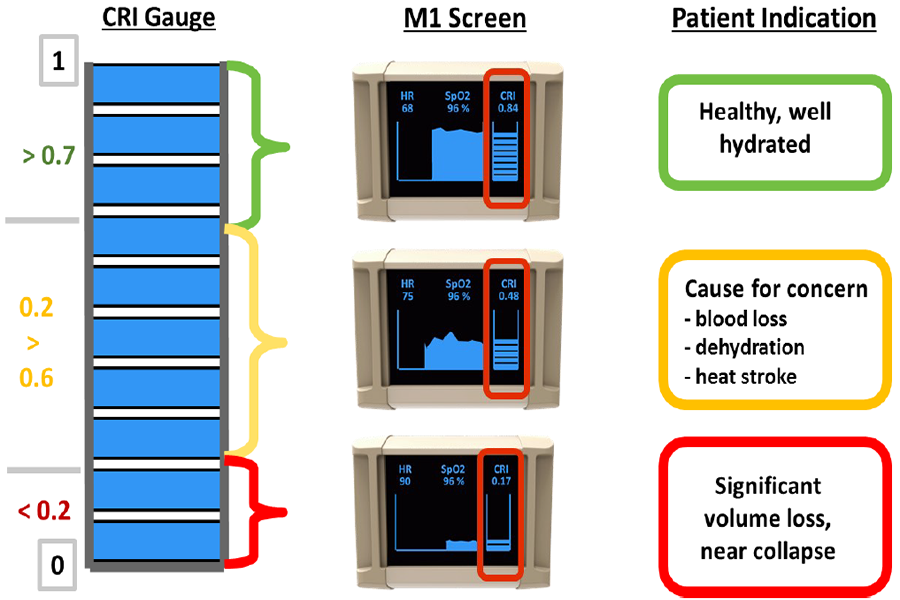
This diagram shows how data from the compensatory reserve index currently on the market correspond to a patient's health status. (Flashback Technologies Photo)
"The CTSA Program funding allowed us to hire computer science graduate students to make faster progress on developing and validating the technology," said Moulton. This pilot support was followed by significant funding from the U.S. Army to develop the device into an easy-to-use monitor. Moulton and Grudic subsequently licensed the intellectual property from CU and launched a start-up company, Flashback Technologies, to commercialize the technology.
To gain approval from the FDA to use the device in hospitals and other care settings, the team had to validate the model in a more real-world scenario. They collaborated with Dr. David MacLeod, at the Duke University School of Medicine Department of Anesthesiology, to conduct moderate human blood-loss studies. The laboratory allowed the researchers to temporarily remove and store up to 20 percent of a healthy volunteer’s blood while monitoring them with the CRI device. The CRI algorithm reliably predicted how close each subject was to collapsing, selecting those who were more sensitive to blood loss and showed symptoms much earlier than the volunteers who were more tolerant of the blood draw. Upon reviewing these data, the FDA approved the CipherOx CRI M1 for broader use.
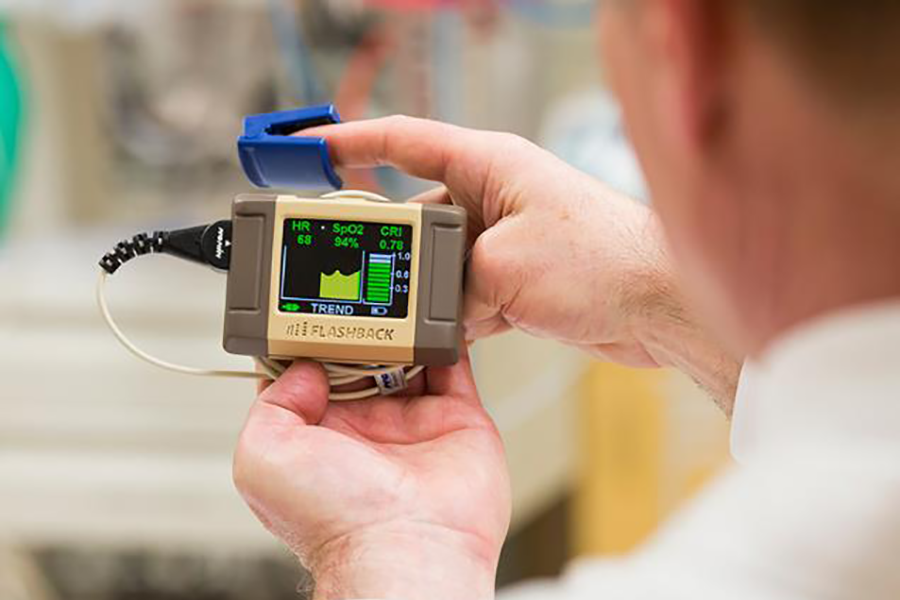
CipherOx CRI M1 with CRI “fuel gauge” to the right and 20-minute trend line to the left. The trend line allows practitioners to determine if their patient is continuing to bleed or if fluid resuscitation measures are effectively refilling the patient’s “fuel tank.” (Children’s Hospital Colorado Photo)
Commercial adoption of the CRI has taken different and interesting turns, according to Moulton. For example, one medical center bought 16 devices to monitor trauma patients being transferred to the hospital by helicopter. Another center plans to use these devices to monitor children who come in with spleen, liver, or other solid-organ injuries instead of drawing routine laboratory studies.
Several centers are interested in monitoring women after childbirth for evidence of postpartum hemorrhage, one of the leading causes of maternal death in the United States. "Too many young, healthy women around the world die every year following childbirth," Moulton said. "We believe CRI will provide a better, continuous noninvasive method to monitor these women and enable practitioners to know how near or far a woman is from collapse. It will enable doctors to intervene earlier, before these women become unstable and are much more difficult to treat."
"It is great to see CTSA Program support at the beginning of a project culminate in a life-saving technology that makes it to market to benefit patients," said Michael G. Kurilla, M.D., Ph.D., director of the NCATS Division of Clinical Innovation. "It demonstrates the catalytic power of NCATS through the CTSA Program."